P.I. Works and Turkcell joined forces under AI4Green to meet targets for increasing the energy efficiency of mobile radio access networks and creating greener, zero-emission networks. Below is the scope of work carried out under AI4Green:
• Proposal of AI-based Network Energy Efficiency and Spectral Efficiency assessment and optimization
• Proposal of AI-based demand response service for the interaction between telecom networks and smart grids
• Solutions for accelerating computing facilities that enable AI in a distributed or centralized architecture
• Solutions for control procedures that optimize network sites’ power consumption and power usage depending on a variety of parameters that could be non-correlated
• Proposal of risk-sensitive optimization for performance management that takes into account the end-to-end path
• Proposal of smart, autonomous, and parameter-free BS (base stations) that learn from the environment and activate relevant energy-saving features when needed, with the optimized parameter settings
• Definition of AI-based network optimization using data (e.g. traffic prediction, resource reallocation, self-healing)
• Definition of KPIs for energy efficiency of network slices and adequate measurement and reporting methods (for energy efficiency standards evolutions)
P.I. Works and Turkcell have fostered a partnership since 2017 with the objective of implementing fully automated network optimization, monitoring, and standardization solutions across the latter’s network. Both teams have collaborated to build new AI-based algorithms that save more energy via smarter predictive carrier-level shutdown actions, predictive sector-level shutdown actions, and crowd-movement modeling that seeks to understand subscriber behavior around hotspot locations, especially during special events. To create these algorithms, three P.I. Works solutions are utilized:
- EXA Network Automation Solution: EXA offers best-in-class, comprehensive AI-based automation capabilities. With its Open API support and flexible automation algorithms, mobile operators can optimize and manage their multi-vendor networks end-to-end, while improving customer experience.
- VantagePM Performance Monitoring Solution: VantagePM, P.I. Works’ next-generation end-to-end performance management solution, is one of the most trusted business intelligence solutions for mobile operators, owing to its self-service, easy-to-use interactive dashboard, reporting, and alarm management capabilities.
- Experia Geolocated Customer Experience Solution: Experia provides integrated geolocation and geo-tagged data analysis and visualization for mobile network management. Experia features unmatched, ad-hoc analysis functionalities, and is able to perform fine-grained root cause analysis and virtual drive tests. Experia provides the means to convert network and customer experience data into actionable and map-based insights, covering a large set of technical, marketing, and customer-care use cases.
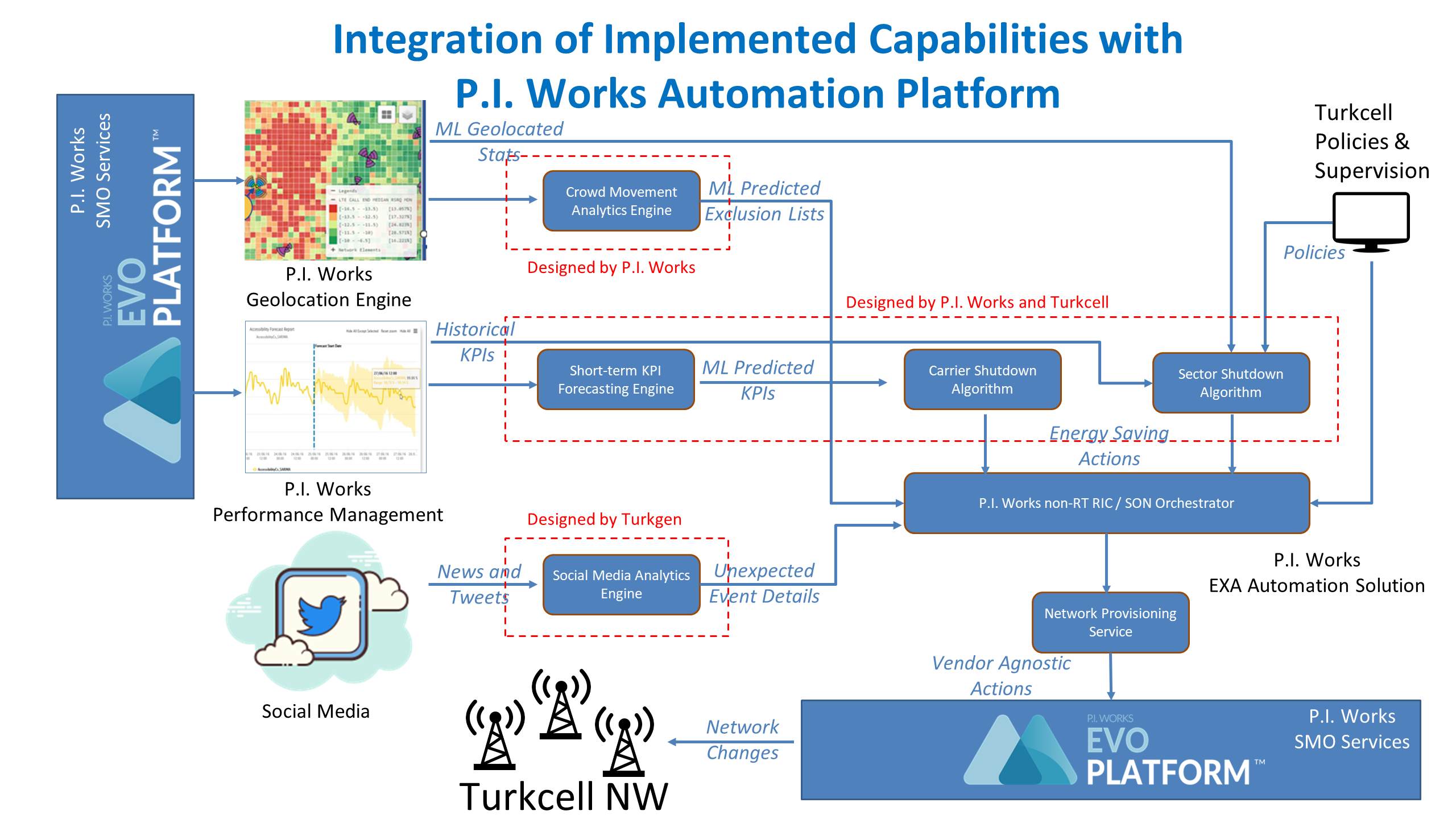
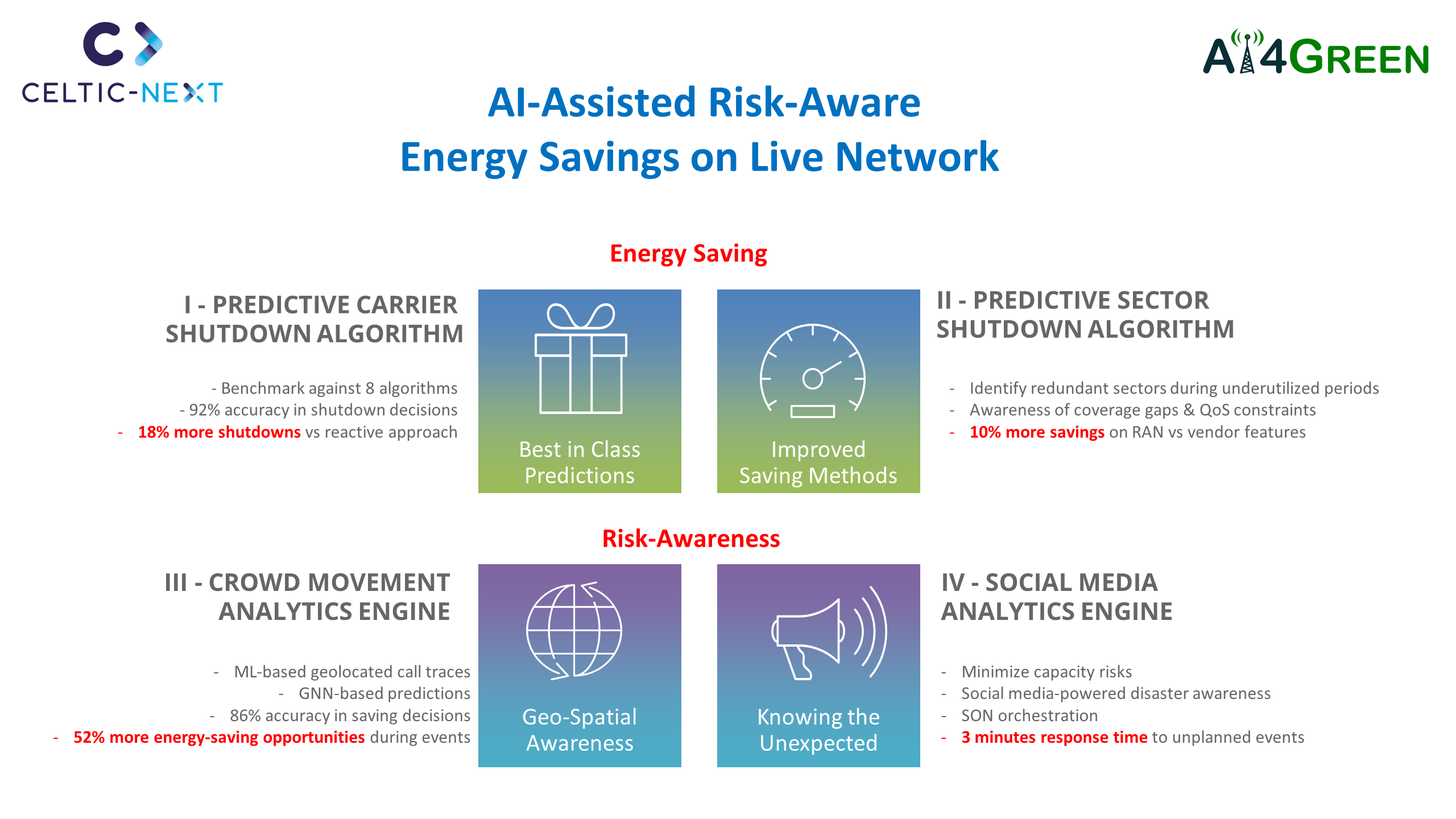
To achieve energy efficiency, an automation application that enables the graceful shutdown of carriers for underutilized sectors based on their frequency clusters was deployed on Turkcell’s RAN (Radio Access Network) and tested in 396 sites across three big cities in Turkey, including the network nodes of two different RAN vendors. Utilization KPIs were predicted for the next two hours and underutilized periods were predicted based on the historical data patterns of each site. ML-based ensemble methods are designed to process autocorrelation values of change-based, sector-specific utilization KPIs collected over multiple weeks of raw data, to enable the short-term prediction of action metrics. Prediction accuracy for utilization KPIs were compared for eight different time series prediction algorithms, including statistical time series methods, ensemble ML models, and deep neural network models, and the most accurate algorithm was implemented on P.I. Works AI Engine service, as an exposed capability of P.I. Works EVO Platform. This predictive carrier shutdown application is built using the API of the EVO Platform and is orchestrated on EXA. As a result, an average of 2.5% more RAN energy savings were achieved, on top of vendor-specific, distributed energy-saving features. The prediction accuracy of the implemented automation application was tested and observed as 92%, based on the classification of predictions into those above or below certain KPI thresholds. The predictive approach was also measured to provide 18.8% more carrier-hour shutdown opportunities, compared to a reactive approach where actions are taken based on measured KPI values only.
Energy Efficiency Focus 2: Predictive Sector-Level Shutdown Algorithm
Having achieved carrier-level energy savings, both teams decided to step up their efforts by implementing sector-level shutdown actions. So, in the second phase of the project, P.I. Works and Turkcell developed predictive algorithms to evaluate the energy savings based on shutting down whole under-utilized sectors. This is more promising in terms of saving energy but is inherently riskier due to potential coverage gap issues. By integrating Experia’s ML-based geolocated subscriber experience logs with EXA, P.I. Works and Turkcell proved that it is possible to mitigate these risks by classifying sectors into those for capacity and coverage purposes, and for different periods during the day, using historical geospatial data analysis. The final predictive sector-level shutdown application was implemented on EXA and deployed in one of Turkey’s most crowded areas, Kadikoy, İstanbul, covering 104 sites by processing billions of measurement logs. Pre-Post benchmarks were recorded based on ML-based geolocation logs, VantagePM performance reports, and Customer Experience Management (CEM) solution reports, to ensure no coverage gaps arise during energy-saving periods. This best-of-breed approach achieved 10% more energy savings on top of vendor-specific, distributed energy-saving features. This corresponds to around 6.5% of total energy savings across the whole network, which in turn corresponds to 1.77 million USD (assuming 0.26 $/kWh) of savings annually.
Risk Awareness Focus 1: Crowd Movement Analytics Engine
Sector-based, energy-saving algorithms leverage predictions derived from historical data to turn on or turn off cells proactively. Such proactive actions for cells, based only on historical data, bring forth risks associated with coverage and overloading on neighbouring sectors due to the unforeseeable movement of crowds. Since these risks are related to crowd movement, modelling the spatio-temporal effects of congestion in one sector on neighbouring sectors can help to mitigate them. In other words, crowd movement can be modelled using the measured key performance indicators (KPI) of cells and the interrelated activity between them, after which the model can be used to eliminate bottleneck risks in highly populated, dynamic areas by instructing energy-saving algorithms to keep certain cells on, where a load increase is expected due to crowd movement.
Planned local live events impact the RAN considerably, during which RIC or SON platforms should be aware of this and orchestrate energy saving and capacity adjustment actions accordingly. For instance, after a football match ends, crowds will disperse to their homes and this movement will result in a heavy load on specific sectors, such as certain hotspot transportation paths and locations. Crowd movement analytic engines aim to learn the patterns of such impactful previous events against normal day patterns to notify the RIC platform or SON orchestrator of potential impact in the future, given a planned live event. Hence, energy-saving algorithms can be stopped in specific sectors and capacity adjustments can be subsequently made.
P.I. Works designed and implemented a crowd-movement modelling engine based on Graph Neural Networks, which was tested during the day of a football match in Fenerbahce Stadium, Kadikoy, İstanbul, across a region covering 1430 cells over a 10 km^2 area. The model takes both performance KPIs from EMS systems collected by the EVO Platform adapters and geolocated customer experience logs from Experia.
To demonstrate the efficacy of this approach, the potential energy savings during a special event where operators generally avoid energy saving altogether is calculated and compared with ground truth. Low-risk energy savings during a live event were measured at 52.5 percent given the trained model predictions, while the maximum possible energy savings were 54 percent given the de facto value. This made it possible for Turkcell to identify which radio sectors are safe to proceed with energy saving and during which periods, by considering the impact of nearby live events rather than stopping energy-saving algorithms due to uncalculated capacity risks. During and after live events, 52% of nearby cells continued to be managed by energy-saving algorithms while those forecasted to experience capacity demand were excluded by EXA automatically.
Risk Awareness Focus 2: Social Media Analytics Engine
P.I. Works, Turkcell and Turkgen also worked on the integration of social media analytics data into P.I. Works’ RIC platform and SON orchestrator to be aware of unplanned events and disasters that are reported or shared on social media. Turkgen contributed to this part of the project with their expertise in natural language processing and understanding, having designed a social media analytics engine which is able to continuously monitor news and social media feed and extract relevant insights from these sources in a well-defined format to P.I. Works’ SON platform via its restful open API. This information is filtered and enriched with cell specific information and relayed to orchestrators and automation applications. For instance, in the case of an unplanned event in a certain location, this occurrence would be identified by Turkgen via their analytics engine and furnished to P.I. Works’ SON platform for estimations on potentially impacted cells and preparation for subsequent scheduled optimization activities.
P.I. Works and Turkcell shared their expertise and experiences with other partners of AI4Green and presented at public events hosted under AI4Green. Both companies closely collaborated and discussed with certain other project partners to achieve these results, such as Turkgen and METU from Turkey, and KTH from Sweden. The project has been commended and deemed excellent by the CELTIC-NEXT committee.
Please get in touch with us at marketing@piworks.net to learn more about our projects and portfolio of offerings.